Every time customers shop, click or engage with retailers, a treasure trove of data accumulates. It fuels business growth, improves customer experiences, and polishes operations when used wisely. Take the example of Amazon. The company invests in sophisticated AI to provide a personalized seller and buyer experience, resulting in a 6.7% year-to-year increase in sales.
Amazon’s data analytics in the supply chain is finely tuned, too. It ensures that products are stocked in the right places at the right times, minimizing delays, and maximizing customer satisfaction.
Read on to learn how data analytics is changing retailers’ work, connection with customers, and competitive advantage. By the end of the article, you’ll know why and how to use analytics in retail projects, what retail software solutions can help you leverage data, and what trends shape the future of the field.
What Is Retail Data Analytics?
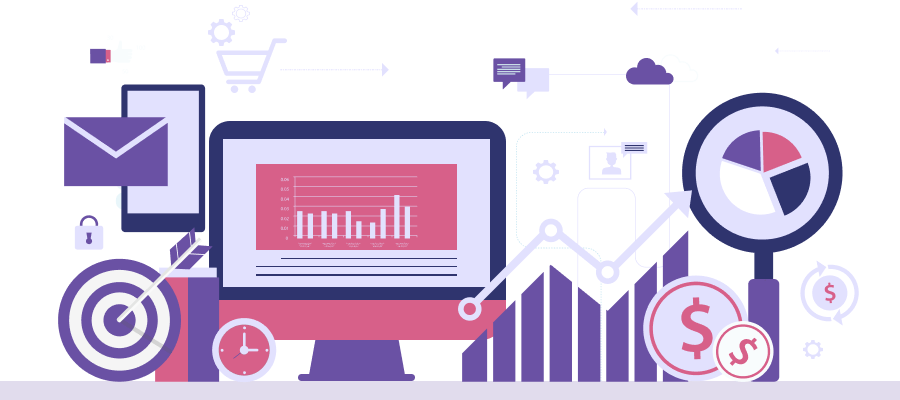
Retail data analytics is the art and science of sifting through the immense amount of information generated by every transaction, click, and interaction in the retail scope. With every purchase, every customer inquiry, and every product return, data is quietly collected in the background.
It is then tirelessly examined to uncover hidden patterns, trends, and valuable insights, all with a single aim — enhancing business performance and sales technologies.
To grasp the full essence of the concept, first, let’s explore the core elements: data types, sources, and methods of its collection and storage.
Types of Data in Retail
Retail data comes in various forms, each offering valuable insights into business performance. Here’s a table summarizing types of data in the retail industry, along with their descriptions, examples, sources, and common use cases:
Type of Data |
Description |
Examples |
Use Cases |
Source/Collection |
---|---|---|---|---|
Transactional | Raw records of sales and purchases | Sales receipts, order histories | Sales analysis, inventory management | Point-of-sale systems, e-commerce platforms |
Customer | Information about individual customers | Names, contact details, purchase history | Customer segmentation, personalization | CRM systems, loyalty programs |
Inventory | Data on stock levels and product availability | Stock levels, SKU details, reorder points | Inventory management, demand forecasting | Inventory management systems |
Market | External data that helps analyze trends | Market reports, competitor pricing | Market analysis, pricing strategies | Market research firms, search engine tools, competitors |
Supplier | Information about suppliers and partners | Supplier details, contract terms | Supplier management, procurement | Supplier databases, contracts |
Employee | Data related to store employees | Payroll records, shift schedules | Workforce management, labor cost analysis | HR systems, time-tracking software |
Sales | Information about product sales | Units sold, revenue, product categories | Sales performance analysis, revenue forecasting | Sales databases, POS systems |
Website Analytics | Data on website visitor behavior | Page views, bounce rates, conversion rates | Website optimization, user experience | Google Analytics, web logs |
Social Media | Data from social media platforms | Likes, shares, comments, user profiles | Social media marketing, brand sentiment | Social media APIs, scraping |
Demographic | Information about customer demographics | Age, gender, income, location | Targeted marketing, customer profiling | Surveys, census data |
Product | Details about retail products | Product descriptions, specifications | Product recommendations, catalog management | Product databases, manufacturers |
Location | Geographical information | Store locations, customer addresses | Store optimization, customer targeting | GPS, geolocation services |
Data Storage
The choice of storage options depends on the specific needs, scale, and resources of the retail business. Companies often employ a combination of these methods to accommodate different data types and ensure accessibility for analysis:
Data lakes are versatile storage solutions. When dealing with diverse data types, including unstructured data like text or images, data lake architecture offers flexibility. It is great for exploration and experimentation.
Data warehouses are centralized repositories designed for structured storage, facilitating easy retrieval and analysis. Consider data warehouse architecture when you have well-structured, business-critical data.
Cloud-based services are scalable and cost-effective, making them a wise choice for businesses looking to manage data remotely and avoid the burden of maintaining physical servers.
On-premises servers are traditional physical servers on-site, offering complete control over data security and management, which is essential for businesses with strict security or compliance requirements.
Hybrid storage is a combination of on-premises and cloud storage for flexibility and redundancy. Use this option to strike a balance between control and scalability.
Distributed databases are designed for handling large datasets across multiple nodes or servers and providing high availability.
In fact, the way you manage data defines how successful your future analytics efforts will be. The data management strategy designed to collect and store retail information will help derive meaningful sales insights from any analysis.
Read more about the Key Differences between Data Lakes and Data Warehouses
BI for Business
Find out the secrets of how business intelligence boosts operations and what BI tools and practices drive data analysis.
Advantages for Retailers
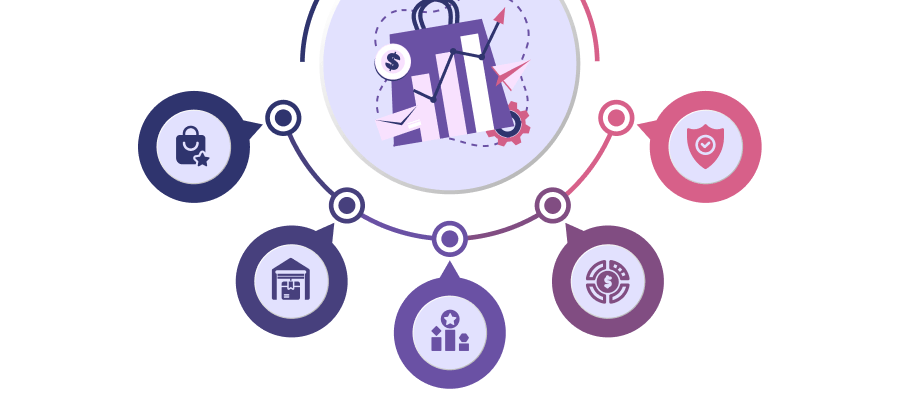
Retail analytics arms managers with data-backed insights into their customers on a personal level. For example, researching shopping habits and using them to optimize retail marketing analytics enhances the overall shopping experience.
But that’s not all.
With analytics, retailers refine their inventory levels, ensuring products are available when and where customers want them. It’s a win-win situation — lower carrying costs for retailers and happier customers who can find what they need.
Data analytics is a strategic technology for business. It reveals market trends and competitor strategies, enabling retailers to adapt and stand out. Besides, analytics ensures efficient resource allocation by identifying top-performing campaigns, leading to a more budget-savvy approach.
Protecting the bottom line is crucial as retailers combat fraud. Advanced analytics detects irregularities, offering security and peace of mind to both businesses and customers.
Whether you’re a small boutique or a global retail giant, the flexibility of a data-driven approach scales to meet your unique needs.
Advanced Retail Data Analytics Techniques
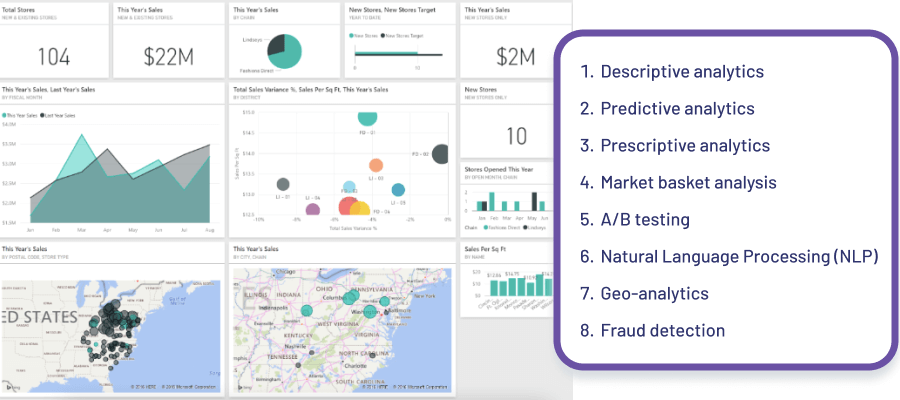
With analytics, you’re not just looking at the past; you’re also predicting the future. We’ll talk about three basic types of analytics: descriptive (telling you what happened), predictive (helping you foresee the future), and prescriptive (giving you smart advice on what to do next).
We’ll also talk about real-life examples like understanding what customers buy together, testing new ideas, understanding customers through language skills, looking at where things happen, and catching bad guys with fraud detection.
1. Descriptive Analytics
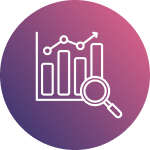
Descriptive analytics is the foundational stage of data analysis in retail, focusing on summarizing and interpreting past statistics to provide insights into what has happened.
Retailers use descriptive analytics to dissect historical sales data, identifying trends and patterns or customer segments. For instance, a clothing retailer may optimize inventory and marketing by recognizing seasonal sales spikes.
As for customer segmentation, an online clothing store might segment customers into categories such as “frequent shoppers,” “budget-conscious buyers,” or “luxury seekers.” This allows retailers to personalize marketing efforts and product recommendations for each group.
2. Predictive Analytics
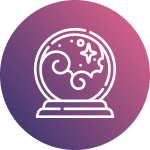
Retailers often use predictive analytics in finance planning, inventory management, and customer behaviors. It fosters improvements in demand forecasting accuracy, inventory turnover, and customer satisfaction.
So, companies apply it to optimize stock levels, avoiding costly overstock or frustrating stockouts. Imagine a grocery chain that employs predictive analytics to foresee increased demand for specific fresh produce due to seasonal trends. This insight enables precise stock ordering, reducing waste and improving profitability.
Retailers also employ it to predict which customers are likely to stop purchasing and take proactive measures to retain them. For example, an online subscription service might use predictive churn analysis to identify at-risk subscribers and offer personalized incentives or discounts to encourage them to stay.
3. Prescriptive Analytics
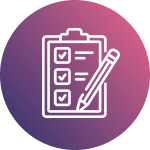
Prescriptive analytics goes beyond predicting future outcomes; it recommends actions to achieve desired results. It is frequently applied for assortment planning or price optimization.
An example might be a fashion boutique using prescriptive analytics to identify the most popular clothing items among its target demographic and adjust its product assortment accordingly. This data analytics approach improves sales and customer satisfaction.
A large supermarket chain might apply prescriptive analytics to optimize pricing for its private-label products. By analyzing various factors, including production costs, competitor prices, and historical sales records, they determine the ideal price points to maximize profitability while offering customers competitive prices.
4. Market Basket Analysis
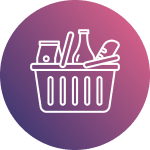
Market basket analysis uncovers associations between products frequently purchased together. For example, in a grocery store, it might reveal that customers who purchase cereal are also likely to buy milk. This insight informs retailers about which items complement each other.
Retailers also utilize market basket analysis to improve the placement of products in stores. Such as a supermarket may position pasta and pasta sauce in close proximity because the analysis shows that customers who buy one often purchase the other. This strategic arrangement boosts cross-selling opportunities.
5. A/B Testing
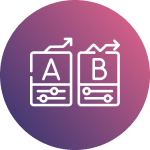
A/B testing, a method of comparing two versions of a webpage or product to determine which one performs better, is another valuable tool in retail marketing analytics.
Conversion rate optimization is a primary goal of A/B testing. Retailers employ it to refine elements like product descriptions, images, and call-to-action buttons to encourage more purchases or sign-ups. A/B testing can reveal which variations result in higher conversion rates, leading to a more streamlined sales process.
6. Natural Language Processing (NLP)
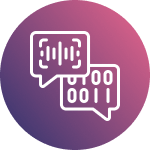
NLP is all about understanding customer sentiment through textual data. Retailers utilize NLP to analyze unstructured data from sources like customer reviews, social media, and customer support interactions. By gauging sentiment and identifying areas for improvement, retailers gain valuable insights into customer preferences and concerns.
NLP aids in product development, too, by providing insights from customer feedback. For example, an electronics retailer can analyze online reviews to identify common issues with a particular product and use this information to inform product improvements. This proactive approach enhances product quality and customer satisfaction.
Today, NLP is one of the most powerful algorithms offered by artificial intelligence in sales.
7. Geo-Analytics
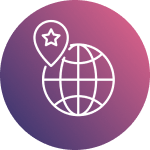
Geo-analytics is the fusion of location-based data and analytics. Retailers employ geo-analytics to make informed business decisions about store expansion and location selection. By scrutinizing demographic information, competitor proximity, and local market conditions, they can identify ideal locations for new stores or assess the performance of existing ones.
8. Fraud Detection
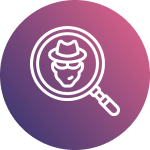
Retailers use fraud detection to protect their financial assets. For instance, they may analyze transaction records to identify unusual purchasing behavior, such as a sudden spike in high-value transactions. Detecting these anomalies early can prevent substantial financial losses.
Effective fraud detection enhances customer trust. When customers know that a retailer takes proactive measures to protect their financial information, they are more likely to engage in transactions and maintain a positive perception of the brand.
Software makes the application of these techniques much easier. Today, software has stepped beyond raw analysis and has introduced a variety of templates adjusted to the industry-specific analytics approaches, including customer-centric retail.
Let’s explore some of the best retail-focused software out there.
Retail Analytics Software
These retail software tools are pretty versatile. They cover everything from handling big data and business intelligence to retail-focused number-crunching and making data look pretty, helping with business decisions.
In a nutshell, they’re your go-to toolkit for a wide range of tasks in the world of analytics.
Software |
How to Use in Retail |
Key Features & Benefits for Retail |
---|---|---|
Tableau | Data visualization for retail |
|
Power BI | Microsoft’s business intelligence platform for retail needs |
|
Google Analytics | Website and e-commerce analytics tool |
|
SAS Retail Analytics | Retail-specific analytics solution |
|
IBM Cognos Analytics | IBM’s business intelligence and reporting for retail analysis |
|
Looker | Data exploration and analytics platform for retail analysis |
|
Alteryx | Data preparation and analytics tool |
|
Python/R with libraries for retail analytics | Programming languages with retail-specific libraries for custom analytics |
|
Big Data Tools (Hadoop/Spark) | Big data analytics for retail stores, distributed data processing platforms |
|
The choice of software depends on the specific needs and preferences of the retailer, with varying pricing models to accommodate different budgets. The enterprise software you utilize already also matters as the integration with it might be very specific.
Challenges in Retail Data Analytics
Analytics comes with its challenges, and addressing these issues is crucial for success in the field.
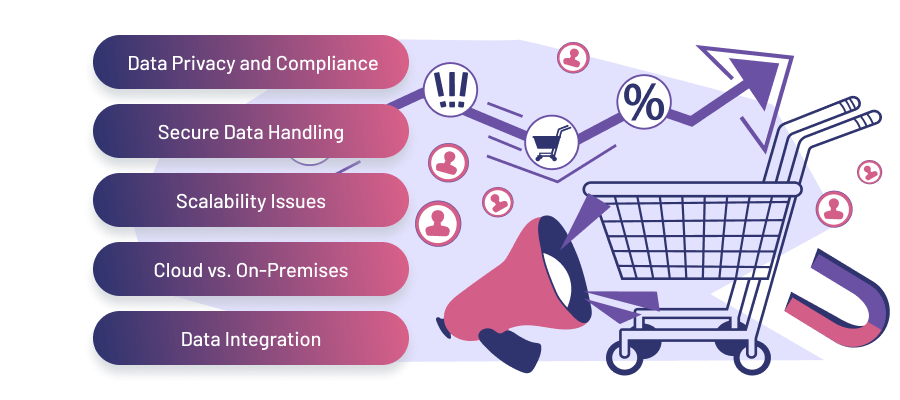
Data Privacy and Compliance
Ensuring data privacy and compliance with regulations like GDPR (General Data Protection Regulation) and CCPA (California Consumer Privacy Act) is key. Retailers must navigate the implications of these regulations to protect customer information and avoid legal consequences.
Secure Data Handling
Maintaining security is a significant challenge in analytics. Retailers need robust mechanisms for secure data handling, safeguarding sensitive information from breaches and unauthorized access.
Scalability Issues
The volume of records in retail can be immense, and managing big data is a common challenge. Retailers must invest in scalable infrastructure and technologies to handle vast amounts of data effectively.
Cloud vs. On-Premises Solutions
Choosing between cloud and on-premises solutions is a critical decision. Each has its advantages and limitations, and retailers need to determine which infrastructure aligns best with their analytics needs.
Data Integration
Data in retail often resides in silos, making it challenging to gain a holistic view. Overcoming data silos and streamlining Extract, Transform, and Load (ETL) processes for data integration are key challenges in achieving a unified analytics environment for a retailer.
So here, we’ve got our fair share of challenges, from privacy and security to dealing with massive amounts of data and deciding whether to go with cloud or on-premises solutions. Plus, there’s the never-ending battle of integrating data from various sources that often live in their own little silos.
But here’s the deal: to tackle these challenges and come out on top, you need the right team of experts by your side. Choosing the right development partner, one that knows the ins and outs of analytics is your secret weapon for success in this data-driven game.
Let’s team up and conquer these challenges together!
Analytics in Retail: Success Stories
These real-world examples illuminate the dynamics of data analytics in retail, showcasing how adaptability, innovation, and customer-centric approaches can drive exceptional success.
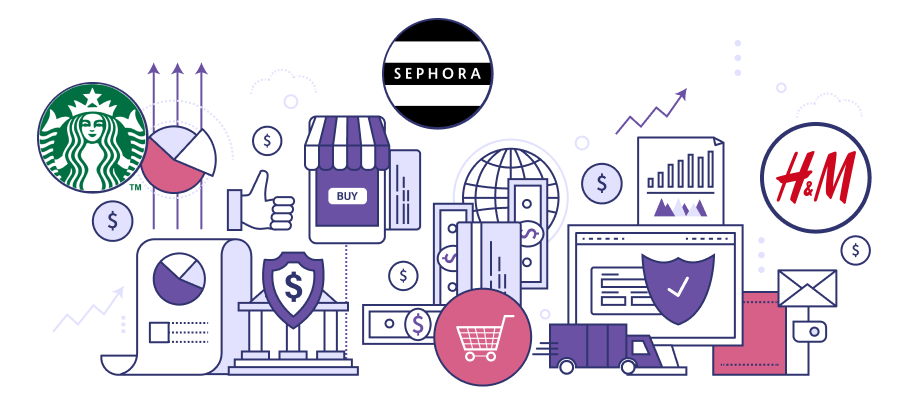
Starbucks’ Customer Loyalty Strategies
Starbucks, renowned for its coffee empire, relies heavily on the potent synergy of analytics to bolster its reign. The linchpin of their success? The thoroughly crafted Starbucks Rewards program.
This loyalty program isn’t merely about earning points; it’s a data goldmine. Every transaction is a data point, and Starbucks meticulously analyzes these to extract invaluable insights. They comprehend that a loyal customer transcends a regular one; they’re fervent advocates for the brand.
Beneath the surface, Starbucks uses this information to refine its analytics strategies. Customer preferences, purchasing habits, and even store foot traffic are scrutinized. This data-driven approach guides decisions ranging from personalized product recommendations to the strategic placement of new stores.
Sephora’s Virtual Artist App
Sephora has redefined the beauty shopping experience with its Virtual Artist app. It uses data gathered from customer interactions to enable virtual makeup trials and style experimentation. Even more, the Virtual Artist app leverages facial recognition technology to analyze skin tones and facial features, recommending makeup products tailored to individual preferences.
This intricate dance of data allows customers to virtually try on different makeup looks, experiment with diverse product combinations, and receive precise recommendations.
In essence, the app serves as a digital beauty advisor, combining customer records with a vast array of cosmetic offerings in the store. Behind the scenes, data analytics powers the magic, enabling customers to discover the perfect shades and products that align harmoniously with their unique styles.
H&M’s Recycling Program
Sustainability is at the forefront of H&M’s initiatives. Their recycling program encourages customers to return old clothes in exchange for discounts on future purchases. The returned clothing is then repurposed, reducing waste and promoting a circular fashion economy.
The program relies on customer data, including purchase histories and recycling preferences, to streamline the recycling journey. Analytics comes into play by helping H&M efficiently manage returned items, identifying recyclable materials, and categorizing them for repurposing.
Explore the Essential Ways to Ensure Sustainability with IIoT
The Future of Data Analytics in the Retail Industry
The future of the field is marked by several key concepts and business intelligence trends driving its evolution:
Real-time insights personalize offers or optimize inventory on the go. For instance, when you shop online and see product recommendations changing as you browse, that’s real-time analytics at play.
AI-powered personalization is like having a virtual shopping assistant who knows your preferences better than you do. Artificial intelligence for business is becoming a norm rather than a trend.
Voice commands like “Hey Siri, order me some new sneakers” or augmented reality (AR) apps that let you virtually try on clothes are making shopping more interactive and convenient. Brands like IKEA allow you to visualize furniture in your home using AR.
Ethical AI is the guardian of data. Companies are adopting ethical artificial intelligence principles to respect privacy and avoid bias in decisions.
The sustainability focus encourages more and more retailers to go green. They’re mindful of sourcing sustainable materials, reducing waste, and adopting eco-friendly practices. For instance, Patagonia’s commitment to sustainability is a great example.
Supply chain transparency using blockchain and IoT technologies is bringing the product’s path to your fingertips. For example, Walmart is using blockchain to trace the journey of mangoes from the farm to the store.
Emotion analytics uses data from facial expressions or voice tones to gauge how you feel. A store may detect when you’re unhappy while shopping and offer assistance or a special deal to turn things around.
Quantum computing analyzes massive datasets in seconds rather than hours. Google is making strides in it.
Collaborative analytics encourages teamwork. It ensures that insights benefit the entire organization, not just a select few.
Robotic Process Automation (RPA) is a robotic helper. It handles repetitive tasks, like data entry, freeing up human employees to focus on more strategic roles. For example, FedEx uses robotic process automation to automate shipment tracking.
Neuromarketing delves into the psychology of consumer behavior. Brands like Coca-Cola use it to create ads that tap into our subconscious desires.
These concepts are making retail software and analytics more efficient, personalized, and responsive. It’s an exciting time to be a shopper!
BI for Business
Find out the secrets of how business intelligence boosts operations and what BI tools and practices drive data analysis.
Planning to Integrate Retail Analytics?
The field is undergoing a transformation, marked by such trends as real-time insights, AI-driven personalization, and the integration of emerging technologies like quantum computing. Amidst these innovations, ethical data practices and sustainability considerations are gaining prominence, reflecting the evolving priorities of retail.
As you contemplate the future of your retail projects, connect with us to get the best out of our expertise. We’ll break it down for you: First, we’ll explore how data analytics integration fits into your retail project. Then, we’ll walk you through the different types of data at your disposal. After that, we’ll lay the groundwork for a data-driven retail approach. Finally, we’ll work together to bring you to a point where you can confidently utilize advanced techniques.