Ever since their first appearance on the market, generative AI technologies have been set to revolutionize how companies do business and interact with clients and stakeholders. However, it’s clear now that integrating generative AI models into production may be connected with certain repercussions.
While the technology’s capacity for good is undeniable, genAI risks and especially the emerging dangers of generative AI deserve much closer scrutiny from experts. For example, although generative AI in healthcare enables physicians to deliver more personalized patient care and make deeply-informed choices, some studies point out the threat of perpetuating algorithmic bias against more vulnerable patient categories if a genAI model was trained on faulty data.
This article uncovers core risks associated with generative AI and large language models (LLMs) and gives a general overview of the current legislative landscape surrounding them.
Possible Risks of Generative AI
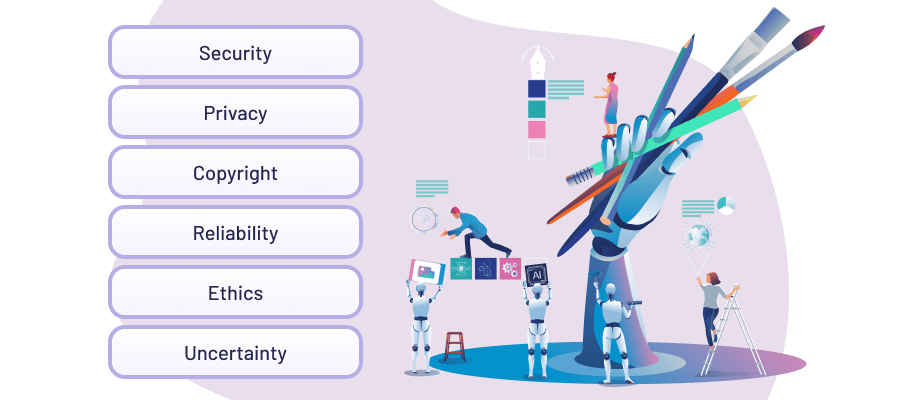
Generative AI offers countless benefits in many spheres. From IT to marketing and even mental health services — genAI models like OpenAI’s ChatGPT, Microsoft’s Bing, or Google’s Gemini can create responses based on user input. At the same time, there are numerous generative AI risks for security, intellectual property rights, and privacy.
“These systems can generate enormous productivity improvements, but they can also be used for harm, either intentional or unintentional.”
— Bret Greenstein, partner, cloud and digital analytics insights, PwC
Generative AI Security Risks
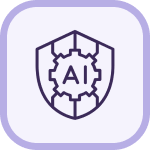
Generative AI risks and security concerns are a multi-level threat that’s not easy to address, especially if an organization-wide integration of genAI models is in progress. On the foundational level, these models are uniquely prone to security breaches due to their complexity and the massive amounts of data used to train them.
Even more so, apart from data poisoning or hacking perpetrated against the model itself, generative AI risks being used for sophisticated phishing campaigns, DDoS attacks, malware production, or unauthorized access to classified data. It is hard to predict the extent of damage done to an enterprise, whose model is hijacked in this fashion.
Another potential liability is the misuse of genAI by corporate employees. Feeding sensitive company data into non-proprietary models through prompts inevitably leads to security breaches, such as code backdoors or data leaks.
As a countermeasure, some companies, such as JPMorgan Chase, restrict the use of third-party generative AI apps, instead choosing to invest into building their own AI tools. For others, extensive employee training on data security can be a sufficient solution.
Copyright and IP Issues
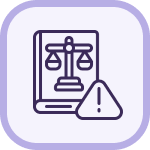
This area of concern presents a two-fold issue. On the one hand, genAI threats in terms of copyright infringement and IP law non-compliance are already familiar but still affect stakeholders in unexpected ways. On the other hand, the question of legal ownership of AI-generated content is a relatively new problem for businesses to tackle.
Due to a lack of transparency in data handling among the developers of generative AI models, there may be copyrighted materials incorporated into training data without the knowledge of the authors. In this way, it could violate the rights of the creators and foster an atmosphere of distrust around genAI. It already happened with StabilityAI and Midjourney, alienating a significant number of artists and even causing a lawsuit.
Some generative AI tool providers reserve the right to train their models on user input, as well as the generated output. This calls into question the ownership of intellectual property and creates ambiguity both for the model’s supplier and the end user. With no clear framework for resolving this type of IP conflict, generative AI issues can lead to massive financial losses for everyone.
Privacy Concerns
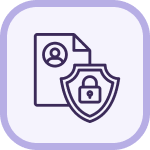
When using or building generative AI models, it is especially important to be attentive to the protection of confidential or personally identifiable information (PII). Failure to comply with personal data protection regulations — such as the integration of personal data into training datasets without consent — can lead to legal liability, reputation damage, and money losses.
Breaches of privacy are particularly dangerous in the spheres of law, medicine, and commerce, where companies sometimes forfeit attorney-client privileges, patient confidentiality agreements, and trade secrets as a result.
An equally troubling implication is the absence of global regulations that establish a readily accessible framework for personal data protection and its removal at the user’s request.
Data and Reliability
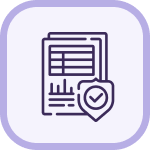
Data is at the core of genAI and therefore its quality is paramount during collection, cleaning, and model training. A number of generative AI risks, namely the reliability and safety of the output, are directly connected with data. In brief, there are several dangers for the user to watch out for:
Hallucinations
Generative AI output is based on a given model’s predictive ability, and this ability, in turn, relies heavily on the training data and conditions. Low-quality datasets and algorithm imperfections may give rise to the so-called “hallucinations” — output content that resembles factual data but is actually pure fabrication.
Using this fake information without serious fact-checking may negatively affect business decisions, particularly in industries where the margin of error is narrow or non-existent. In a famous case, Google’s shares lost $100 billion after an unsatisfactory demo introduction of Bard back in 2023, where it made a single factual mistake.
Systemic Bias
One more outcome of not cleaning the training data carefully is algorithmic bias. Models fed with datasets that lack inclusion or diverse representation show partiality in their output, normalizing skewed perspectives and social injustice. Notoriously, an experiment run on Stable Diffusion AI showed racial and socio-economic bias displayed by the text-to-image generator.
Distribution of Harmful Content
The “garbage in, garbage out” concept in machine learning is relevant to heightening generative AI risks, associated with polluted data making it into the model’s training datasets.
This is especially true for models that are capable of independent data mining on the Internet, like ChatGPT-4, but do not discern which data is beneficial and which is harmful. Thus, they allow explicit or degrading content to manifest in the output due to the information they consume.
The viral spread of news about AI’s harmful behavior may cause not only lawsuits but reputation losses — Microsoft’s Bing had several incidents that users classified as attempts at manipulation or gaslighting.
Misinformation
As a powerful information-producing tool, genAI is instrumental in facilitating learning and discovery. Especially, while it aids highly-qualified human professionals in what is known as “human expert augmentation”. However, the same power may be used in global misinformation campaigns to spread inaccurate or fake content at scale or foster negative user experiences.
Fraudulent Materials
Numerous attempts at fraud with the help of generative AI are now known in many spheres, including academia, law, medicine, and the arts.
A study in May 2023 uncovered generative AI’s high potential for writing fraudulent academic articles. They are virtually indistinguishable from real ones, complete with fake references that are very hard to spot even for professionals. Apart from very real legal consequences, this issue leads to a lack of trust and stability for workers and industry leaders alike.
Ethical Considerations
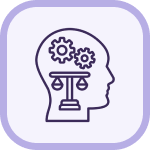
Together with the risks of unlawful use, there are other ethical concerns companies have to face in connection with genAI and the workforce. As jobs get cut at increasing rates due to the development of artificial intelligence, more and more skilled professionals fear for their future.
Both massive layoffs and the reluctance of companies to make new hires introduce instability in the job market. Last autumn, for example, Stack Overflow let go of approximately 28% of its employees to replace them with genAI coding assistants. In this opposition of ethics and profit, the only fair solution benefitting all stakeholders is to help employees develop generative AI skills through training opportunities so that nobody is left behind.
Uncharted Territory Risks
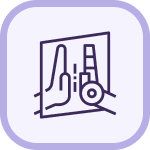
The more powerful a generative AI model is, the more it resembles a “black box” in terms of interpretability and predictability. As a result, a number of genAI risks remain unexplored, creating a latent powder-keg situation. Not wishing to face these risks or lacking proper consultant support, enterprises become resistant to change and miss vital opportunities to adopt the latest technologies.
On the global market, the inability to adapt to the current needs of the industry by identifying and integrating genAI may be even more challenging than any potential problems that may arise.
GenAI for Business
Watch our webinar to uncover how to integrate GenAI for improved productivity and decisions.
Regulatory Challenges in Generative AI Use
With the rapid development and propagation of genAI tools, the drive to regulate this sphere and mitigate generative AI anxieties is gaining momentum. Meanwhile, a fragmented legislative landscape and lack of a unified approach to AI governance are creating hurdles for enterprises eager to introduce generative AI into their work pipelines.
Here are some of the most prominent challenges to date.
Undefined Legal Landscape
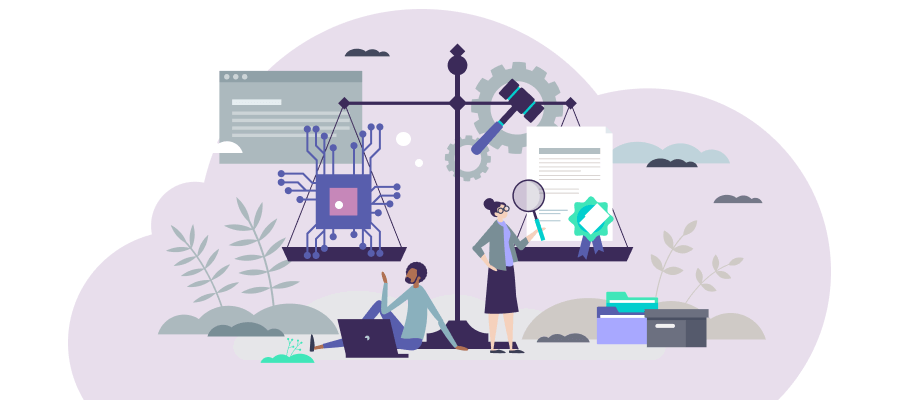
Looking at the current state of AI regulations, two main challenges are evident. First, lawmakers may struggle to produce comprehensive legislation for possible misuse and risk levels of AI systems. Second, reaching global cooperation is not always easy.
Only a handful of countries and organizations, such as the EU, South Korea, and Canada, have proposed or produced binding legislation to control AI use. On the contrary, others have set up non–binding policies and voluntary agreements, or in some cases, no regulations at all, e.g. India, Saudi Arabia, etc.
Such a lack of harmonized legislative efforts creates threatening divergence for global companies to contend with, which makes an equal integration of generative AI in all markets practically impossible.
At the same time, this situation brings opportunities for engaged stakeholders to participate in policy-making. By championing compliance they can gain tangible long-term benefits. An example of this is the cooperation between tech giants like OpenAI, Meta, Microsoft, Google, and others with the US administration to voluntarily produce guardrails, mitigating generative AI risks.
Current and Future Legislation
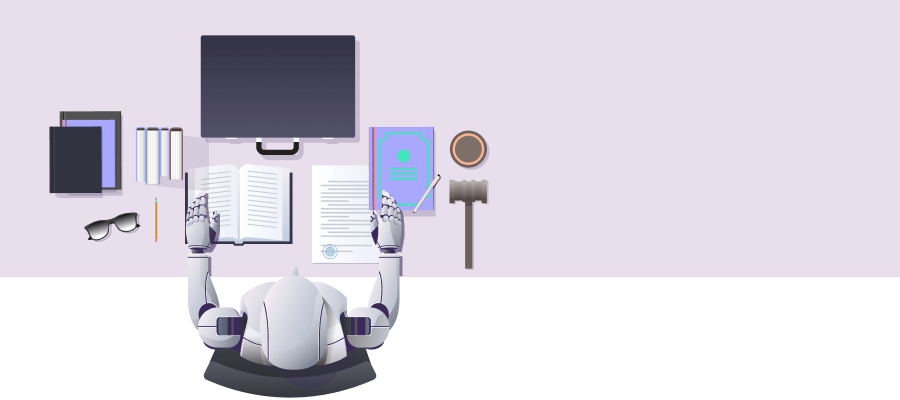
As we’ve mentioned, at the moment, only a few countries and wider political bodies have a developed framework to tackle the challenges of generative AI on a legislative level. With most acts, such as Canada’s Artificial Intelligence and Data Act, still pending approval, the EU’s AI act appears to be the closest to becoming a reality.
On December 9, 2023, the European Parliament reached a provisional agreement with the EU Council on the contents of the document, which will now have to be formally adopted by both the European Parliament and Council to become EU law.
The Act offers flexible guidelines for handling AI technologies based on the level of risk they can pose and interacts with the GDPR and product safety legislation. As a document outlining a broad range of unacceptable or dangerous AI use scenarios, it may become a reference for a unified approach to regulating artificial intelligence globally.
In the USA, however, the situation is more nuanced, with at least 25 states, Puerto Rico, and the District of Columbia introducing AI legislation, and 18 states and Puerto Rico enacting it.
At the same time, there have been no definitive steps to regulate this sphere on the federal level, apart from the October 2023 executive order, issued by Joe Biden and aimed at boosting AI safety and security. According to the document, a range of measures will contribute to the protection of consumer privacy, preventing discrimination, evaluating the presence of AI in healthcare, and creating guidelines for utilizing generative AI in the judicial system.
The latter is especially welcome due to the prolific use of LLMs by some courthouses. As a result, judges may face convincing legal documents that are full of problematic AI “hallucinations” or simply non-compliant with a certain state’s regulations.
Other future motions toward establishing a solid AI regulation framework include the US collaborating with international partners on unifying AI standards worldwide, creating a new AI safety institute, and introducing a Blueprint for an AI Bill of Rights.
One more initiative was announced by the Biden administration last year. It focuses on reformulating existing statutes to apply to the use of AI in perpetrating traditional crimes.
In essence, it brings together several regulatory bodies concerned with protecting consumer rights, such as the FTC, EEOC, Department of Justice (DOJ), and Consumer Financial Protection Board (CFPB) — all in an effort to mitigate possible damage from the misuse of artificial intelligence, including generative AI.
Risk Mitigation and Compliance
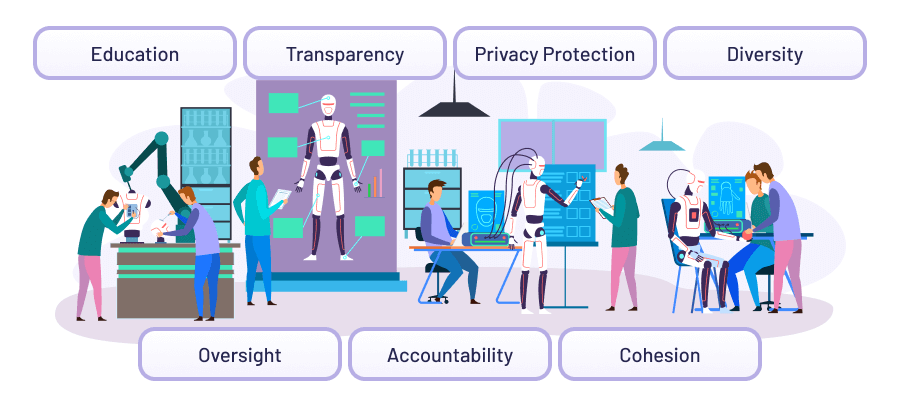
So far, worldwide attempts at regulating AI on the legislative level have mainly concentrated on privacy, diversity, transparency, oversight, and accountability. On top of that, there’s a focus on the technical and environmental safety of these technologies.
To ensure future compliance, enterprises should develop long-term cohesive AI strategies, geared toward reliable data and model management. This includes performance monitoring on all integration stages and developing robust cybersecurity policies.
As far as people are concerned, stakeholders are advised to pursue employee education commitments, including guidelines and training for safe generative AI interactions, PII protection, productivity, and ethics.
Another option is to assign AI oversight to a consulting expert or committee liaising with senior-level executives, such as Chief Technology Officer, Chief Legal Officer, Chief Data Officer, and others. This will help harmonize AI controls and keep them in line with the enterprise’s core values.
Without a company-wide concerted effort, awareness and willingness to abide by the existing and upcoming legislation, it’s easy to get tangled in class-action lawsuits and other legal issues. This was the case of GitHub Copilot, where its creators were accused of violating the copyright of a vast number of creators.
Minimizing Missed Opportunities
Despite all the threats of genAI outlined above, the biggest challenge companies may face in the years to come is the failure to keep up with its rapid development. With no responsible and efficient generative AI implementation, enterprises stand to lose a lot in terms of creative output, innovation, and performance.
If you are struggling with introducing generative artificial intelligence into your business processes, do not hesitate to reach out to us. Velvetech has solid experience in navigating the changing landscape of AI technologies and can successfully advise you even in most intricate cases. Let’s shape the future of generative AI integration together and create an ultimate experience for your company and clients.