Digital information has proliferated into all areas of the modern world, especially the business sector. Naturally, companies are looking for optimal ways of storing and leveraging it for operational success.
As a result, there is a rising demand for data lake and data warehouse implementation. In fact, the market size of both systems is forecast to grow significantly in the coming years. Specifically, the data lakes market is expected to reach $17.6 billion by 2026, while the data warehousing one should hit the $51.18 billion mark by 2028.
“For companies to build a competitive edge—or even to maintain parity, they will need a new approach to defining, implementing, and integrating their data stacks.”
— McKinsey
Despite this popularity, some corporate leaders may be unsure about which solution is best suited for their company. Hence, in today’s post, we’ll discuss the difference between a data lake and a data warehouse so that you can get an idea about what each one can do for your firm.
Understanding Data Lakes and Data Warehouses
First, let’s get the terminology out of the way so that we are all on the same page. While data lakes and data warehouses are both used for storing big data in the enterprise software ecosystem, they are not identical. As such, it’s important to first understand what benefits each one can deliver.
Data Lake
A data lake is a centralized repository that allows companies to store structured and unstructured digital information that they collect from various sources. In essence, it’s an enormous pool of data that is kept in a raw state until it is retrieved for processing.
Despite the lack of structure, IT teams can run various types of data analysis on the myriads of information from the data lake. Whether you want to run predictive analytics or train your machine learning algorithms — uncovering useful insights gets easier with this technology.
Find out about Predictive Analytics in Insurance
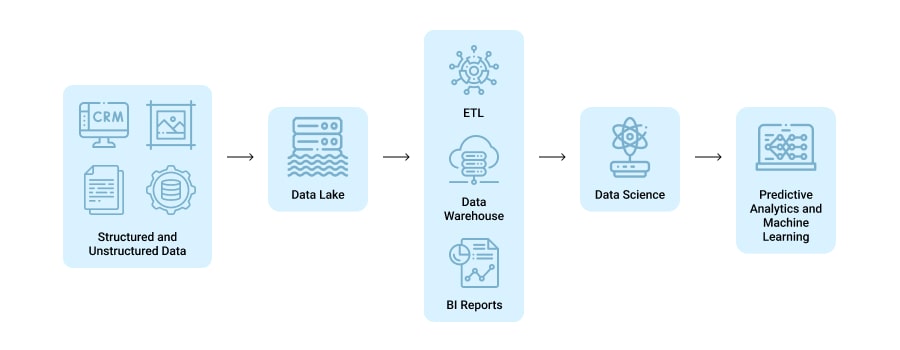
As you can imagine, data lake benefits are vast, but these are the most valuable ones:
- Simplified data management
- Increased operational efficiency
- Reduced costs related to data storage
- Enhanced data security and governance
Despite these advantages, it’s important to remember that when raw data is stored with little oversight, the system can quickly turn into a “data swamp”.
So, when implementing data lakes, don’t forget to define proper methods of cataloging and securing the information you collect. That way, it’ll be easier to make sense of it and find the needed elements when the time comes.
Data Warehouse
On the other hand, we’ve got data warehouses. These systems serve as repositories for structured operational data that’s already been processed for specific analytical purposes.
A data warehouse follows a “schema-on-write data model”, which basically means that a source’s digital information must fit into a predefined structure prior to entering a warehouse. Naturally, this requires the team to spend more time on planning and forming a true understanding of what the platform will be used for.
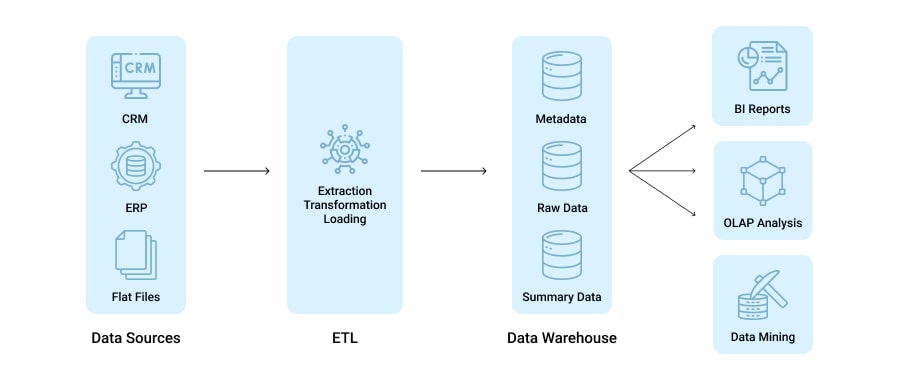
Given the distinct nature of data warehouses, their benefits differ from that of data lakes. With the former, you can expect to observe the following:
- Improvement in data quality and consistency
- Accurate business intelligence
- Ability to run historical data analysis
- Informed decision-making
As you can see, unlike data lakes, data warehouses are created to manage structured data for clearly defined use cases. So, if you aren’t sure what you’d like to use certain digital information for — there’s no need to implement it into a data warehouse.
BI for Business
Find out the secrets of how business intelligence boosts operations and what BI tools and practices drive data analysis.
7 Differences Between a Data Lake and a Data Warehouse
When discussing data lakes vs data warehouses, there are several key differentiating factors that clearly separate the two technologies. Below, we’ll go through each one so that by the end of the article, you can be clear on what each system is good for.
Data Lake |
Data Warehouse |
|
---|---|---|
Data Structure |
Raw, unprocessed data | Structured data |
Data Purpose |
Undetermined | Determined |
Data Processing |
ELT | ETL |
End Users |
Data scientists and engineers | Data and business analysts, department managers |
Size |
Can be very large and require bigger storage capacity | Typically smaller in size than data lakes |
Flexibility |
Flexible, accessible, easy to update | Complicated to update and make changes |
Costs |
Fairly inexpensive | Can be quite costly, depending on requirements |
Data Structure
As we’ve briefly mentioned above, data lakes and data warehouses work with differently structured data. While the former stores all kinds of digital information, including that which is raw and unprocessed, the latter is solely focused on structured data that can fit into a relational database schema.
A data lake can store information from all kinds of sources — IoT-powered devices, social media platforms, web-based solutions, mobile apps, and others. Of course, some of this data may be structured, but that’s not always the case, and since it’s directly ingested into the system from the source — no structuring takes place in advance.
Check out how we built a system for gathering real-time data in a Cloud Database for Cold Chain Monitoring
On the other hand, with a warehouse, it’s necessary to first clean the data and organize it so that it fits into the predefined schema. So, the structure of the stored data is completely different between the two platforms.
Data Purpose
In a data lake, the purpose of the stored digital information typically isn’t determined. Businesses simply ingest any potentially relevant data they collect into the system without yet knowing how it will be used in the future.
Conversely, the purpose of a data warehouse is clearly identified prior to implementing it so that only the necessary details are collected. For example, if you know that you need a centralized platform to automate data processing related to insurance policy management specifically, a data warehouse is what you need, not a data lake.
Take a look at how Velvetech Automated Data Processing for a Recruiting Firm
Data Processing
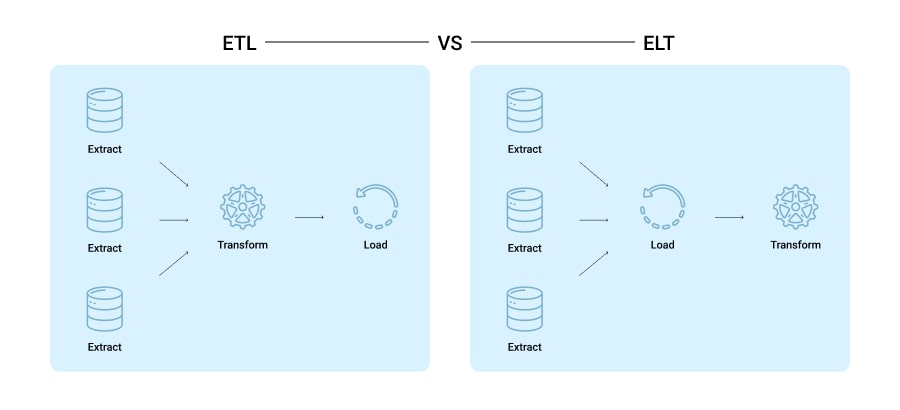
As you may have already gathered, data processing occurs differently in data lakes and data warehouses. In the case of the former, the ELT (Extract, Load, Transform) process is usually followed. Here, data is extracted from the source, loaded into the data lake, and is transformed only when needed.
On the contrary, data warehouses tend to rely on the ETL (Extract, Transform, Load) process during which data is scrubbed and structured after extraction and only then loaded into the warehouse for business analytics.
End Users
Due to the unstructured nature of data in a data lake, it can be difficult to navigate for business professionals that aren’t familiar with the IT sphere. As such, data lakes are most often used by data scientists and data engineers who can set up and maintain them, deploy machine learning algorithms, or integrate them into data pipelines.
Insights from data warehouses are much easier to understand and are thus used by managers and business analysts who are familiar with the organizational area that is being analyzed.
Size
Since data warehouses are rather selective with the data they work with, they tend to be a lot smaller in size than data lakes, which store pretty much any information that is relevant to your company.
So, if you’re convinced that a data lake will serve your business well — you’ll need plenty of storage space to accommodate all the details you’ll be collecting.
Flexibility
When we speak about flexibility and accessibility, data lakes are certainly more susceptible to change. You see, since they have little structure, accessing the digital information and incorporating any changes is quite seamless. As long as the person doing it is skilled in working with data of course.
On the other hand, a data warehouse’s structure is worked on extensively during development. As a result, it is quite complex and, although adaptable to change, any post-deployment manipulation requires extensive developer resources and time.
Costs
Data lakes are usually less costly than data warehouses, in spite of their larger size. This is due to the fact that the latter requires thorough planning and analysis while the former only needs to be set up and isn’t too costly to maintain.
When you’re planning your next project, software development costs are certainly at the top of your mind. However, don’t pick data lakes solely based on this factor. Like we said, everything depends on the goals you want to achieve, and trying to save right now may end up costing you more in the long run.
Data Lake vs. Data Warehouse: Which Is Right for Your Organization?
Data lakes and data warehouses are often used in modern organizations that are looking to leverage the digital information they have access to for lasting business growth. Although the two technologies are clearly different, they can actually work together and be complementary when a company wants to have a strong data management strategy in place.
Velvetech’s specialists are skilled in delivering data lake and data warehousing services that cater to the unique needs of any client. So, if you want help implementing either of these solutions — don’t hesitate to reach out. We’ll be happy to guide you on your data strategy journey.